Predictive medicine
Delivering the right treatments, at the right time, every time to the right person.
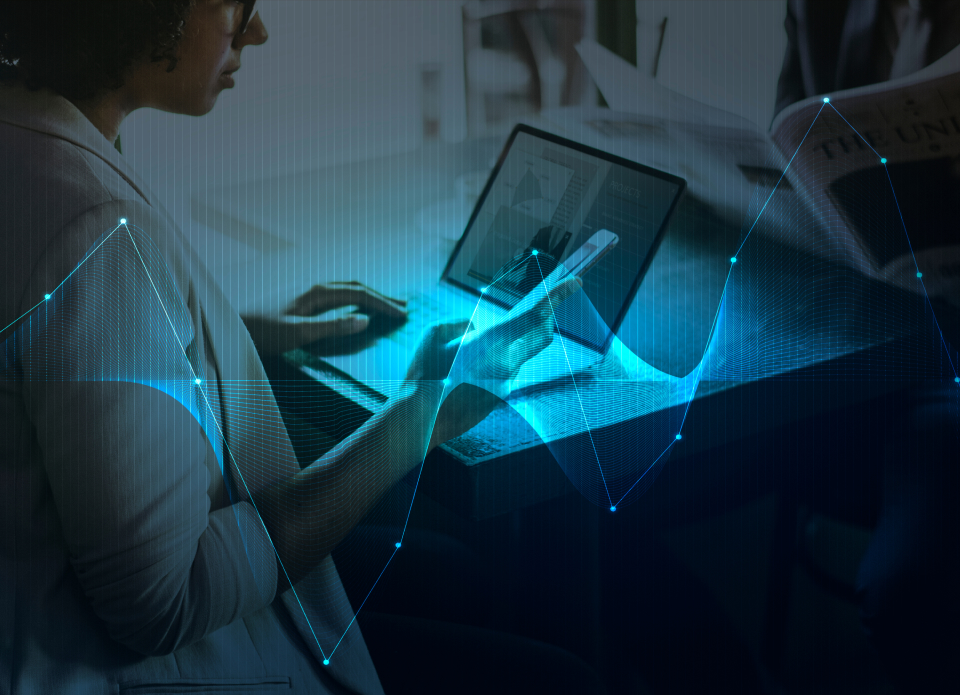
Machine learning approaches
Machine Learning approaches in Predictive Medicine using Electronic Health Records data
- EHR Data
- Artificial intelligence
- Pathology: Screening, Prediction Explainability
- Specialist
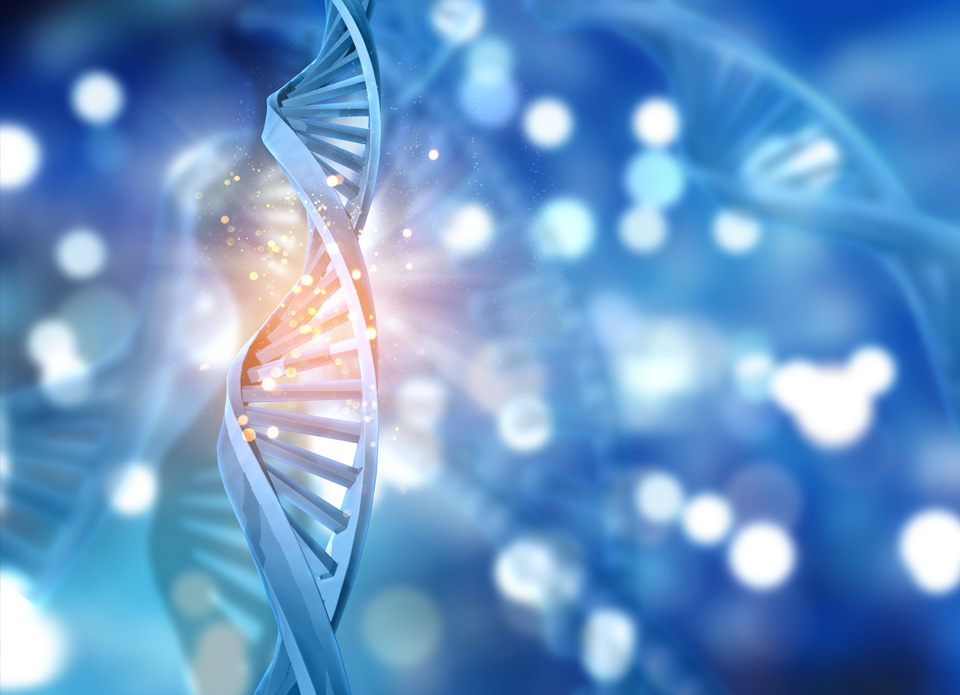
Predictive precision Medicine
The traditional medicine is One-size-fits all, the predictive medicine is preventive, proactive and precise. These healthcare ecosystems of predictive precision medicine are not yet being used at a large scale. The removal of several obstacles could accelerate this transformation process.
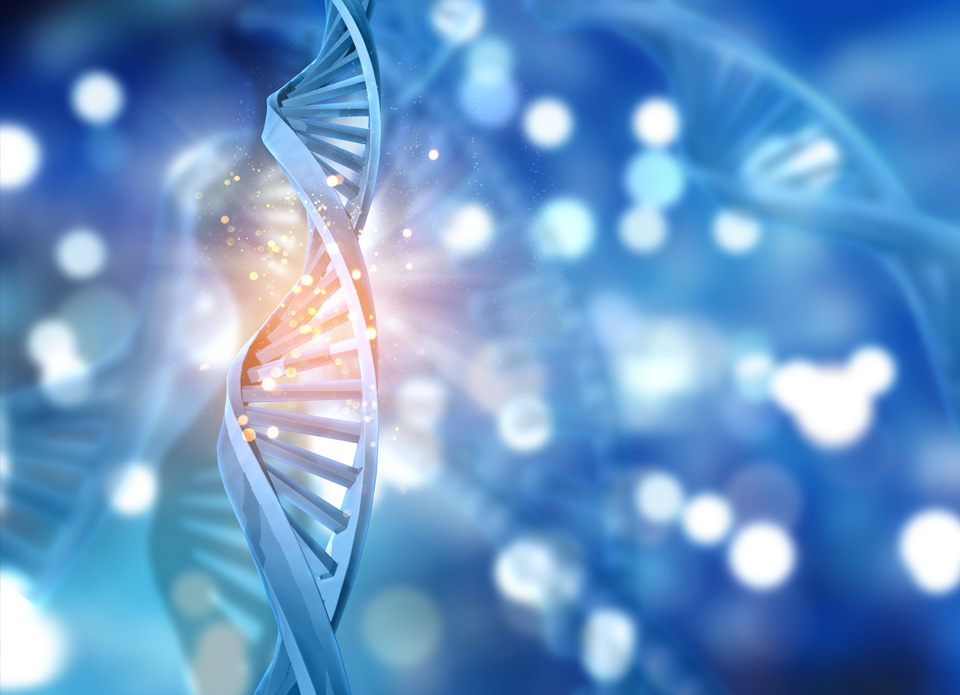
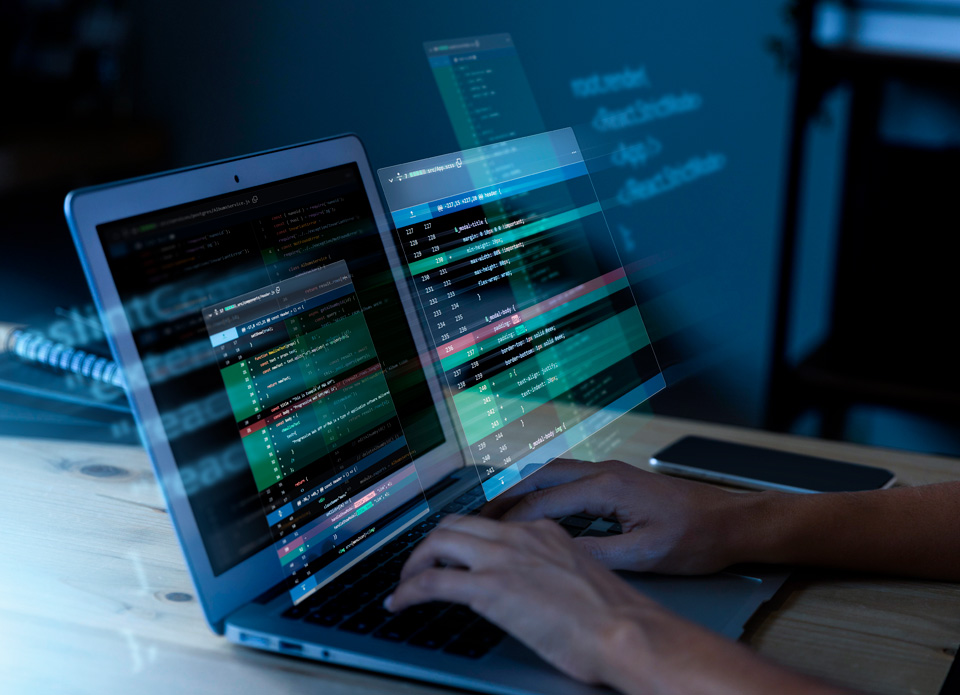
The obstacles
The removal of several obstacles could accelerate this transformation process:
- The first obstacle is the preprocessing stage (i.e., data cleaning, preparation, and standardization);
- The second obstacle is the ongoing need to build layers of abstraction that permit various users to interact with ML frameworks at their own knowledge level;
- The third obstacle is the commonization of components.User-friendly ML frameworks should be designed as modular blocks and selected depending on the objective of the clinical task.
Problem statement
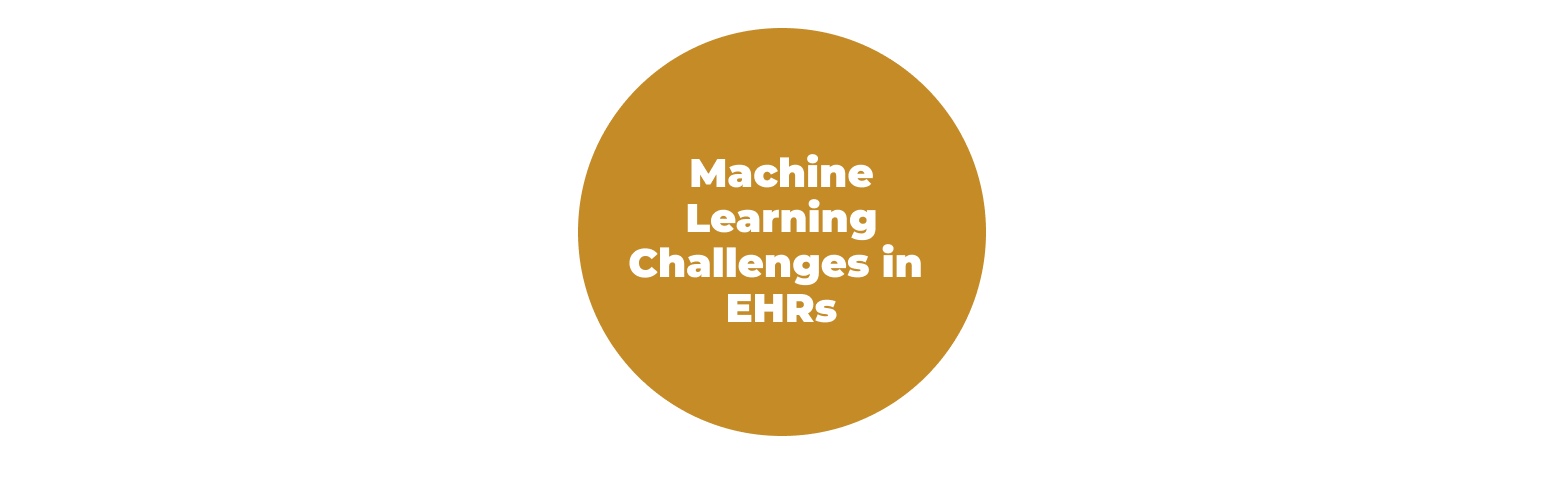
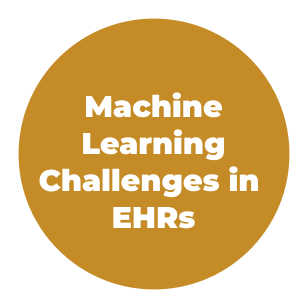
Temporal ambiguity
Model Interpreatibility
Generalization
High dimensional data
Unbalanced setting
Sparse Labeling
These healthcare ecosystems of predictive precision medicine are not yet being used at a large scale.
- High-dimensional & heterogeneous data were managed during the preprocessing stage (i.e., features selection, standardization, outliers detection);
- Unbalanced setting was managed by adopting specific optimization metrics and/or optimal thresholds for the posterior probabilities of the decision function;
- Sparse labeling of the predictors was managed with standard static data imputation techniques (i.e, extra-values, mean, median, K-Nearest Neighbors (KNN)), while sparse labeling of the targets was managed by proposing semi-supervised learning (SSL) techniques;
- Temporal ambiguity was managed by proposing different experimental configurations (i.e., time-invariant, stacked-temporal, Multiple Instance Learning (MIL), Multi-Task Learning (MTL) with temporal relatedness/constraints);
- Interpretability/explainability of the results was managed offering always a features importance ranking of the most discriminative predictors to clinically understand the outcome of the ML model;
- Generalization was managed by adopting regularization strategies.